It's On Us
As artificial intelligence becomes mainstream, who will guide it?
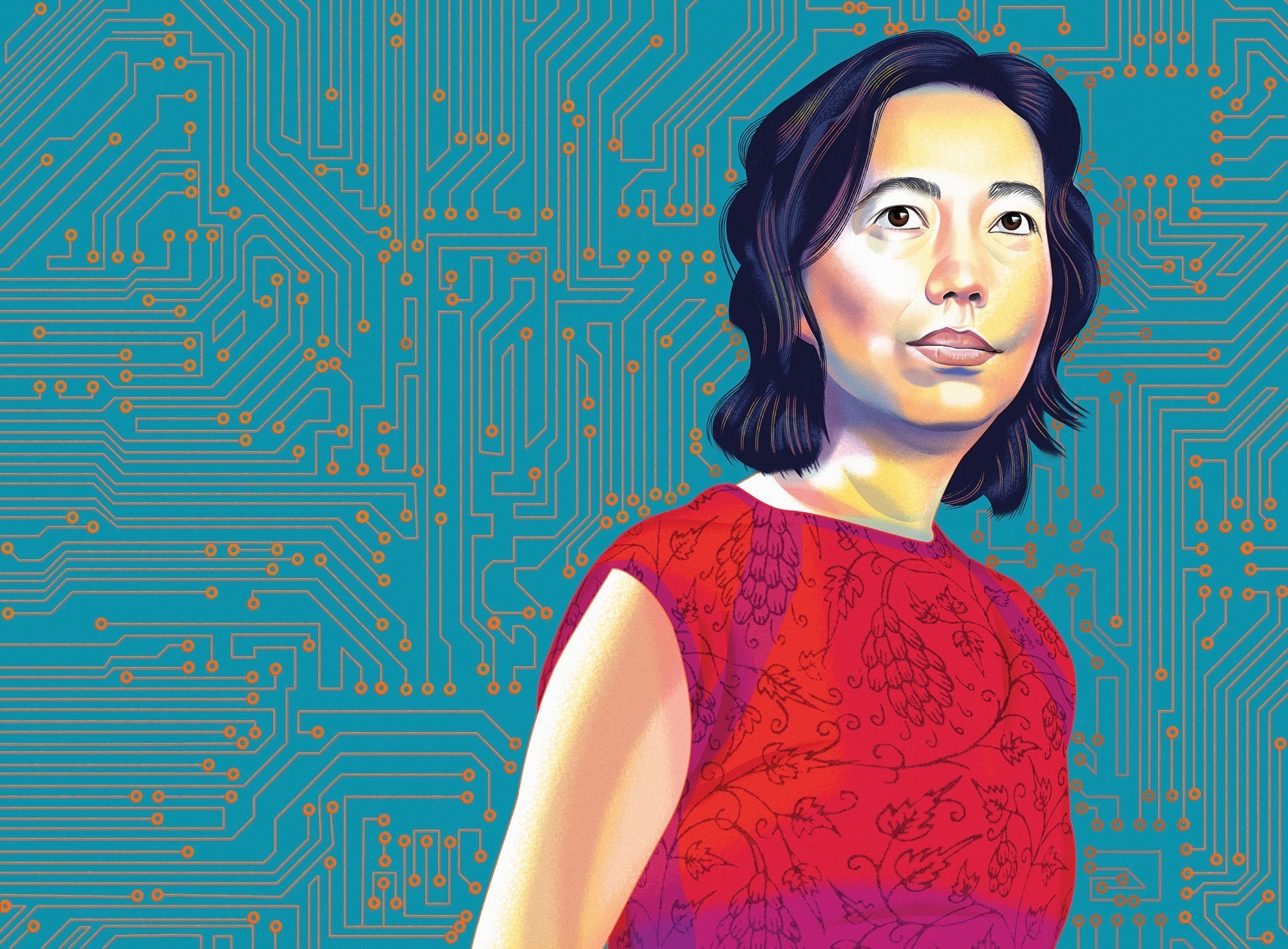
Nigel Buchanan
Artificial intelligence is a trending topic, but few have the bona fides of Fei-Fei Li (PhD ’05). The former A.I. leader and chief scientist for Google Cloud—where she oversaw research in A.I. and machine learning as well as led Google A.I.’s China Center—Li now directs the Stanford Artificial Intelligence Lab and co-directs the Stanford Vision and Learning Lab. Who better to answer our burning questions: Will A.I. replace our jobs? What else will it take over? And who will oversee its development—both technologically and ethically? Here she offers her thoughts on the world of A.I., its promising yet controversial future, and why empathy should play a major role in its design.
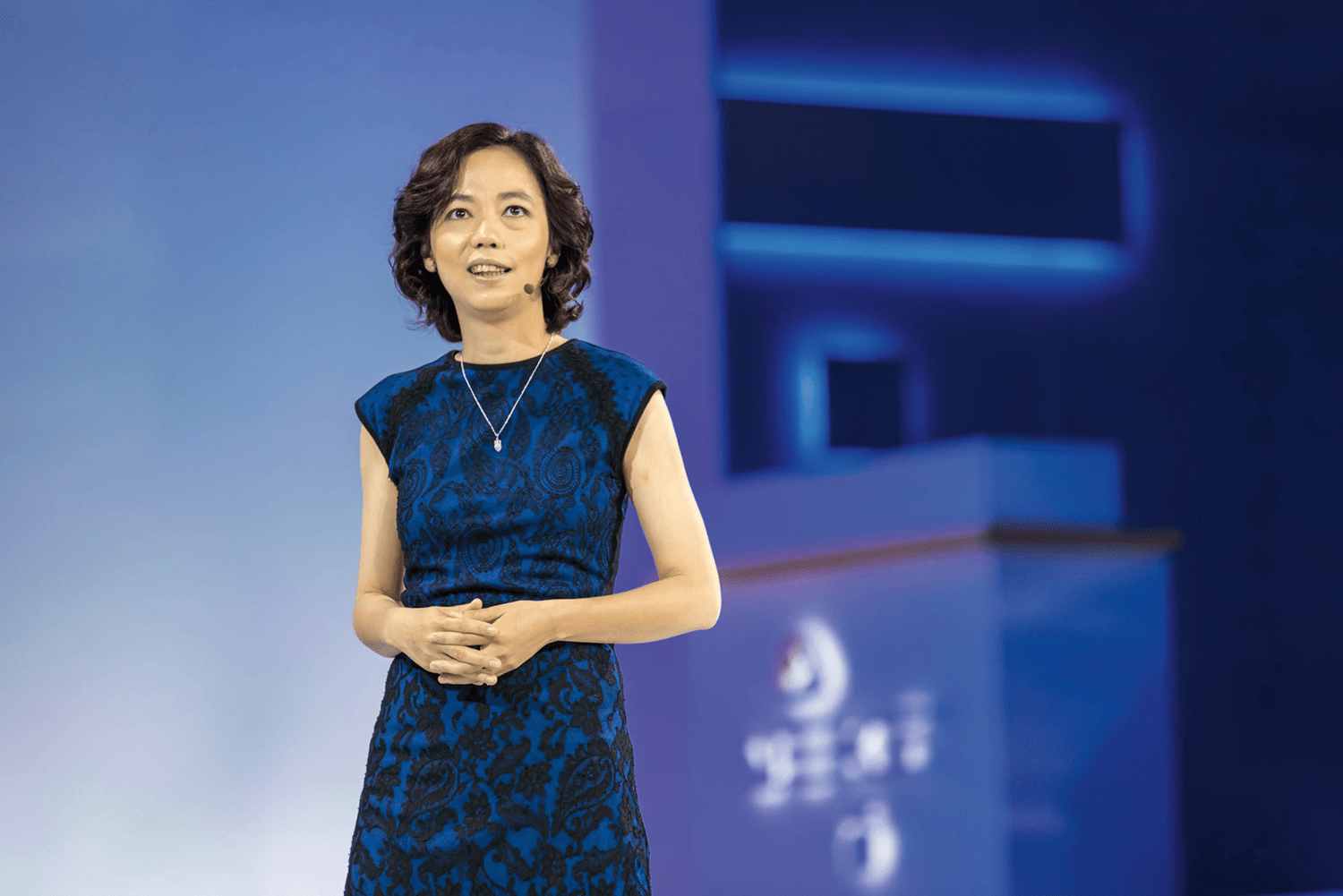
You did a presentation at the Grace Hopper Celebration in 2017 wearing a T-shirt that read “A.I. will change the world. Who will change A.I.?” So, who will change A.I.?
As we see artificial intelligence impacting the real world, it’s no longer a niche computer science, technical field. Policymakers, business leaders, educators, social scientists—they all need to take part and guide the future of A.I.
Also, as a technical field, A.I. thoroughly lacks diversity. It lacks women and underrepresented minorities. I’m the co-founder and chairperson of the nonprofit AI4ALL. We’re committed to education. We’re committed to diversity, especially starting with high school students. It’s unthinkable that such an important technology that will influence humanity has such an imbalance in terms of the representation of people taking part.
A.I. doesn’t belong to a niche group of people. A.I. doesn’t belong to Silicon Valley. A.I. doesn’t belong to tech giants or STEM or A.I. labs. A.I. belongs to everyone, and we want everyone to participate in changing it.
You talk a lot about “human-centered A.I.”—the idea that A.I. and humanity can co-exist peacefully. What does “human-centered A.I.” mean from your perspective?
Human-centered A.I. is made up of three major pillars that guide the thinking of the future of artificial intelligence. The first pillar revolves around human-inspired, next-generation A.I. technology. There’s so much excitement about A.I., but it’s a very recent field—only about 60 years old. It’s actually still very narrow in terms of its capabilities. So we should continue to develop A.I. with inspiration from human intelligence, especially crossing fields like brain, cognitive, and behavioral sciences.
The second pillar is focused on a single word, “enhance”—as opposed to “replace.” There’s a lot of worry, and rightfully so, that automation is at odds with human jobs. And I think that’s a really important topic. But I think the opportunity as a technology is that there’s so much more we can do to use A.I. to enhance human capabilities, to augment them, to take humans out of harm’s way, to make human productivity better and more efficient. Take healthcare, for example. I just spent months with my mother in a hospital after a major surgery. It was an interesting experience because I’ve been doing research in A.I. in healthcare for six years. And as a patient’s family, it became real to me. Doctors, nurses, and clinicians need time to be with patients. Now we can aim assistive technology at that need. And we can use A.I. to take humans out of harm’s way in rescue situations. We can use A.I. to enhance personalized education. We can use A.I. to make more fair and more efficient decisions.
And then the third pillar is what I call “A.I. social impact.” It’s critical that we recognize that all of human society will be impacted by this technology. That means we’ve got to get the social scientists, the economists, the legal scholars, the ethicists, the historians, the philosophers—all of them—to participate in understanding what kind of impact this technology will have, what kind of policy recommendations we should make, how we should guide this technology so it is not biased, and how we can protect privacy in the A.I. era. These issues cannot possibly be resolved by just technologists. It requires a societal dialogue and effort.
How can we address some of those challenges in the design of A.I.?
In the past few years, it’s been recognized that machines are at risk of having biased results when the data input is not fair. And this could cause great harm and unfairness to people’s lives. So machine-learning fairness is a major research area in academia. I know my colleagues at Stanford, at Princeton, and at Google are all working on this, and there are mathematical algorithmic approaches being explored. There’s always policy recommendations that are being discussed.
As I age, I also realize how important empathy is.
We’re technologists, but we’re humans first, and we should have empathy. And we should design our technology with that in mind.
Your work has been described as “democratizing A.I. and machine learning for everyone.” How are you trying to achieve this?
Mostly through my work at Google Cloud, where I continue to work as a consultant. Cloud is a huge computing platform that can deliver the power of computing services to billions of people, literally, through businesses. And we develop A.I. products that could be served through this Cloud platform.
Machine learning is still so new that very few developers in the business world know how to code it. So most of the solutions have been through APIs where you don’t have to code, and you’re just putting your input in and getting the result. For example, image recognition: You’re putting an image into Google’s vision API, and you get labels like “dogs” and “trees” and “cars.” But there’s a huge gap between generic labels like dogs and trees to specific brands of shoes or brands of houses or furniture. We’ve got a lot of wildlife preservation biologists, for example, wanting to just look at different animals, and they need what we call custom models. But they don’t have the talent to code them. So lowering the bar for businesses to create these customer models is a huge step toward democratizing A.I.
You’ve talked about the impact on businesses with this one product. What’s the potential for how A.I. might alter our economy globally?
A.I.-related services and products have had explosive growth. They’re making billions of dollars and are projected to grow exponentially. Whether you’re in e-commerce or healthcare or manufacturing or financial services or media entertainment or agriculture, you need to deliver better services and products to customers—and that process needs better data analytics, better decision-making, better personalization, better recommendations. All this will be using A.I. So every industry that shapes human lives will more or less be impacted by A.I.
What are your hopes for the future of A.I.?
It’s so hard to ask a scientist to predict the future, but I want to see more innovations in A.I. in healthcare. I want to make sure that as A.I. reaches people’s lives, that it is fair, transparent, and that it really makes a positive impact in healthcare delivery, in solving the challenges of our aging society, and in delivering healthcare to more people by making it more accessible.
“A.I. doesn’t belong to a niche group of people. A.I. doesn’t belong to Silicon Valley. A.I. doesn’t belong to tech giants or STEM or A.I. labs. A.I. belongs to everyone, and we want everyone to participate in changing it.”
– Fei-Fei Li (PhD ’05)
How do we ensure that there’s earned public trust in the advances we’re making in A.I.?
A lot of effort has to be made. Machine-learning algorithms need to be fair, need to be transparent, need to be clearly explained and understood—the dialogue needs to continue. Google rolled out A.I. principles as a step toward that, but it’s a multidimensional effort. Earning public trust in A.I. is not a one-time thing. It is continuous and long term—as in start now and never stop. And it needs to be in the minds of business leaders as much as the technologists themselves.
What are the problems you’re working on now? And what are you hoping to tackle in the next 10, 15, 20 years?
I’m particularly excited to look at the healthcare delivery process. I know A.I. and machine learning have started to make waves in big-data healthcare, such as radiology, medical imaging, and healthcare records. As a career vision scientist, I actually took a different perspective and looked at the care delivery in the physical space of healthcare, like hospitals, clinics, senior homes, ICUs, and so on. And once you look at that environment and all the human activity of clinician-to-patient care, there are two major issues. One is that healthcare delivery can be highly inefficient. A nurse in the ICU does more than 100 different tasks, and it’s exhausting. Some of these tasks—charting, endless typing into a computer—takes time away from patient care. And this kind of inefficiency can be assisted by A.I. technology. Voice input, for example.
Another issue in healthcare is human error. Hospital-acquired infection alone kills more than 90,000 people every year in the United States—three times as many people killed by car accidents. And how do we monitor that process? How do we give feedback to our clinicians who should be practicing proper hand hygiene?
A.I. can be explored to create smart hospitals and smart senior homes. It’s a very recent exploration, but my team has been at the forefront of this. We just published an opinion piece in the New England Journal of Medicine and are publishing machine learning and healthcare research papers on this topic. And there is just so much to be done in that area.
Fei-Fei Li on AI and Machine Learning