AI for All
Startup founder Zehra Cataltepe on how humanity will shape the era of AI
by Maureen Harmon
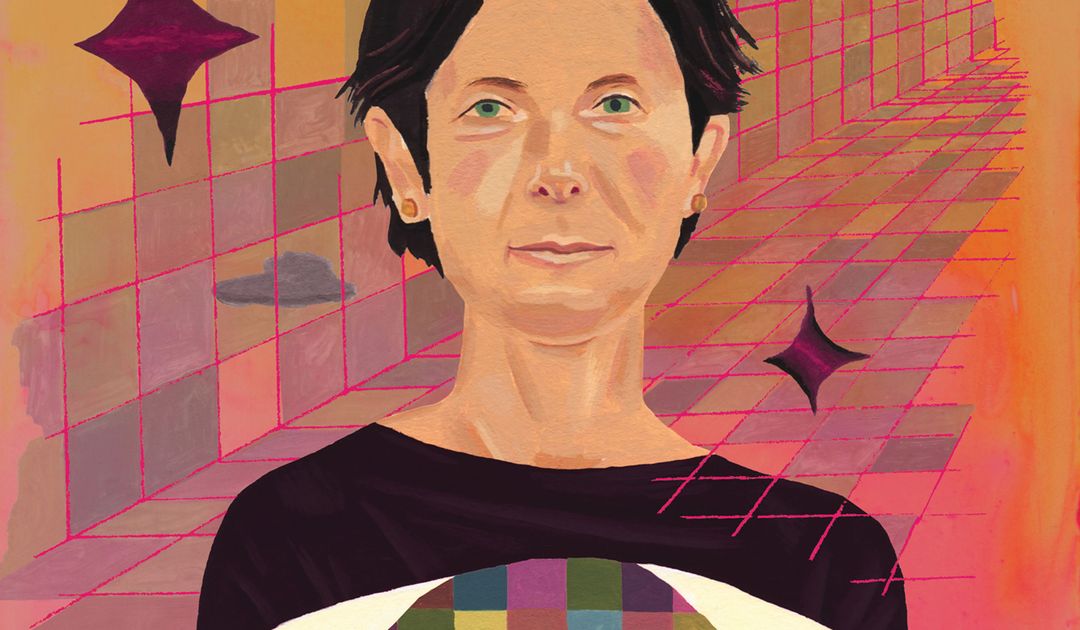
Illustration by Juliette Borda
In 2017, Zehra Cataltepe, PhD (MS ’94, PhD ’98) launched TAZI AI to create AI products and tools that are easy to understand and operate—and open the technology up to professionals in every field. This accessibility is key, says Cataltepe, not only to create technology that is better for the end user, but also to combat bias in its programming. “Right now,” says Cataltepe, “my concern is: Whose voice will the AI hear? Will it only hear those rich or tech-savvy enough to adopt it and use it?” In this conversation with Techer, Cataltepe talks about the role we all play in the future of AI.
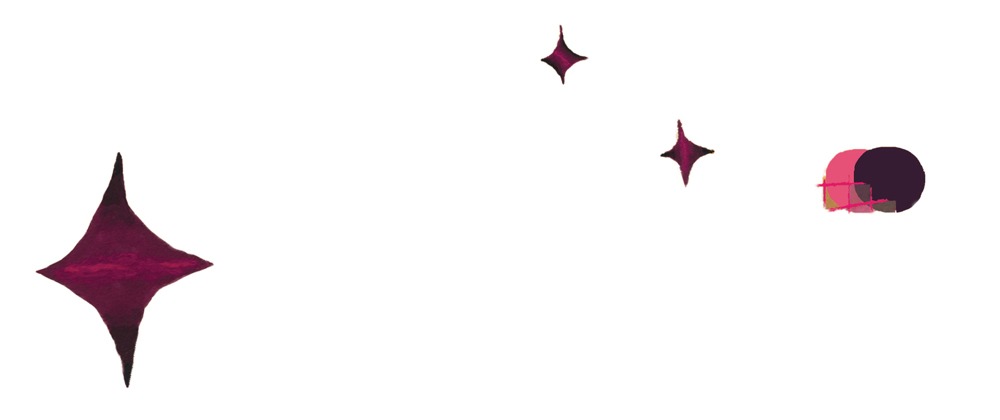
You were an academic before launching TAZI AI. Why did you make the leap to entrepreneur?
Zehra Cataltepe: After I graduated, I did a short postdoc, worked at a startup company, and then started working for Siemens Corporate Research. My work there was in creating AI-based predictive maintenance models to assist mechanical engineers. Our goal was to automatically predict faults and defects that could cost a company millions. The speed of AI-based predictions could significantly cut risk and maintenance expenses. However, I eventually switched to academia to do more independent research. The schedule also allowed me to be at home with my kids outside the work day. While in academia, I also consulted for industry research projects, wrote papers, and filed patents, but the time came when I had had enough of papers and projects. I wanted to see AI in industry again—as a product in real action.
What was the problem you were trying to solve with TAZI AI?
ZC: The usual AI methodology is that somebody gives you a dataset which shows the past behavior of their system, and you build an AI model based on that dataset. What happens in real life is that, after you’ve got your data, time passes and the world changes. You put your model into production and suddenly realize that it doesn’t work as well as it worked in training. Consider how the domain experts, like the mechanical engineers I mentioned earlier, operate. They don’t look at only the dataset. They have accumulated tens of years of experience on the specific problem you’re trying to solve, as well as similar problems. Their knowledge is a lot more valuable than the mere sum of what is in the dataset. The other issue is bias and other problems with models that only become apparent in longer-term deployment. Right now, only data scientists know how to correct these issues in AI. A business owner has to find the issue and explain it to a data scientist, who then has to fix it. That’s a difficult path.
My cofounder and husband, Tanju, came up with the idea of training AI while it is in production. Instead of waiting for it to fail and then retraining, we need the AI to be continuously learning and continuously updating itself, even when in production. This is how we humans operate. When we work at our jobs, we use a lot more than our school years, or our “training sets.” We should also establish a symbiotic relationship between AI and human domain experts. AI can learn from human expertise and humans can learn from AI’s ability to digest billions of data points immediately.
Think of AI as an employee in your company. You will do good work together if, and only if, that employee adapts to your needs. That is, only if you can understand how they’re operating, and they, in turn, can respond to your feedback. If one of these things doesn’t happen, you are not going to be able to work with that employee. So I see AI models as newbie apprentices in our organizations, which make us more capable and our jobs and lives easier.
What are the biggest challenges to combating bias in AI?
ZC: Everybody concentrates on bias in the data. But the bias in the data arises from bias in society. If you do nothing about that, your AI will be shaped by the biases in your data.
While people mostly account for the direct effects of variables such as age, gender, or race, bias can manifest via variables indirectly affected by or correlated with these variables. For example, ZIP codes and credit scores can be highly correlated with race. While there are regulations aiming to address these kinds of biases, there might be other, more complex biases that are not as evident.
A requirement of making sure that AI is really not biased against certain groups is making sure that the creators of AI represent a diverse range of human experiences and backgrounds. As an example, the creators of AI are currently mostly data scientists or engineers—and these people are mostly men. Even if they mean well, they are liable to not notice bias against women.
Another concern is accessibility. Our historical data contains bias, just like history itself. But if you make AI understandable and easy to use and update, then the population that is using and updating it will have the chance to correct biases in that AI. Most recent AI models (including the ones we have at TAZI) learn both from the data that we feed them and from the deployment-time behavior of a human in their operational loop. We thus need to make sure everyone can give feedback to AI to drive its future behavior. This requires both making AI products easier to use and update, and training people for specific AI related tasks. Just as we learned to drive bikes, cars, trucks, planes to take us places, future generations will need to learn how to operate different AI models.
You have said that it is possible to fix bias. How long will it take?
ZC: As human beings, we are quite adaptable. If something works really well for us, we adopt it fast. Cell phones were adopted quickly and are continuously tweaked to make them better for the consumer. We will continue to make AI products more and more usable. I believe that some regulation is required, and is already on its way. The US usually has a good balance between innovation and protecting its people. I hope we will see this also for AI regulation.
Most importantly, we’ll introduce more people to AI. Today, one third of businesses use AI. I’m guessing that will become most businesses within five to ten years. And the more people and businesses use AI, the more we will be debugging it and the better it will be trained to correct for bias.
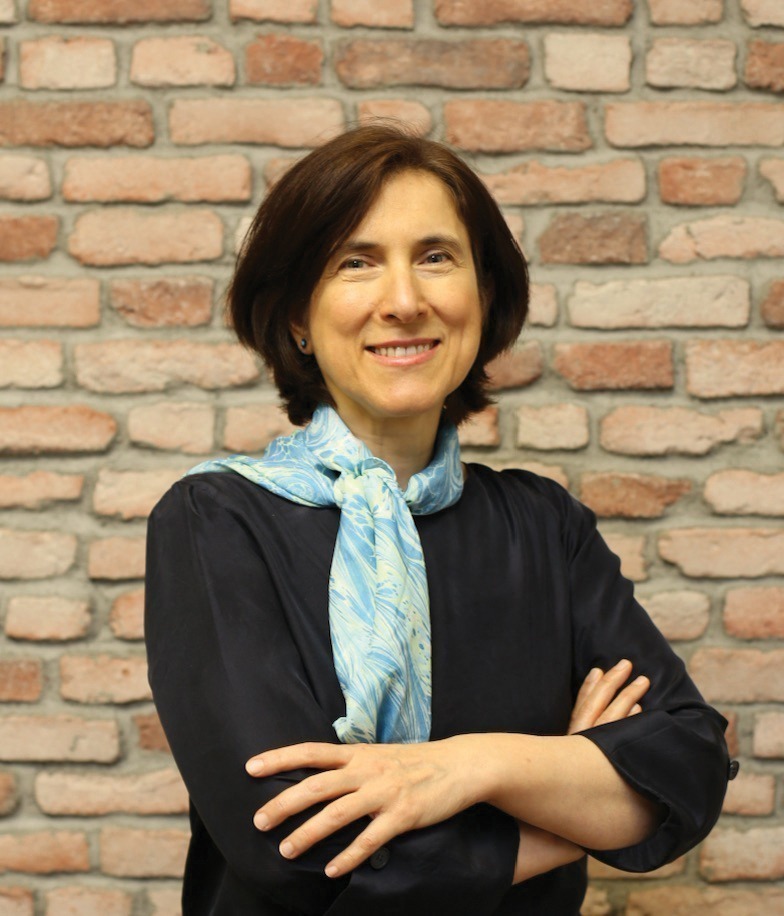